Showing top 0 results 0 results found
Showing top 0 results 0 results found
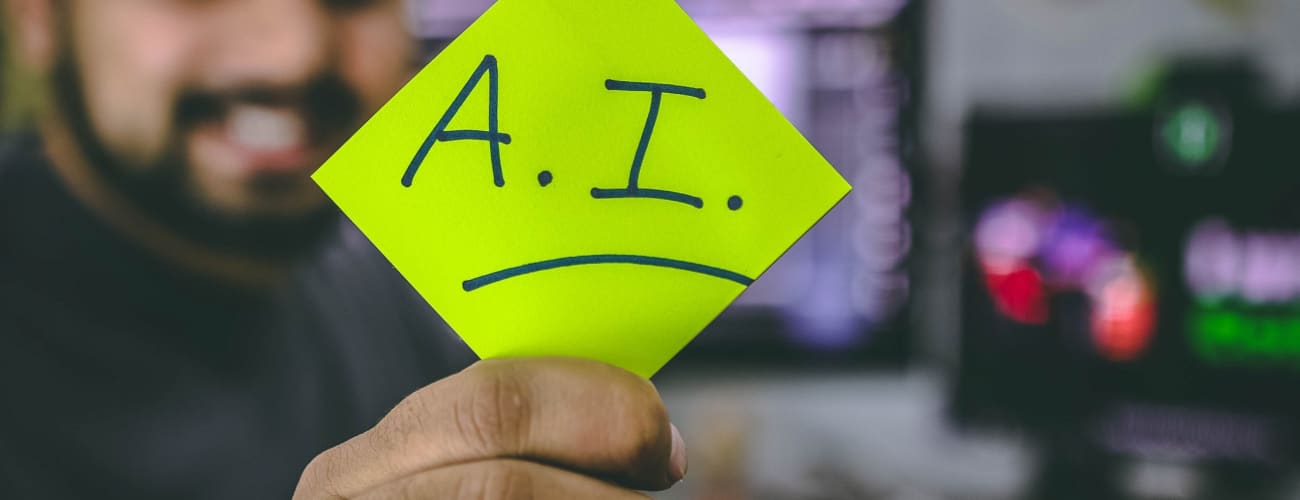
Artificial Intelligence is a hot topic. However, not everyone knows how to put it to work. According to MIT study, companies that actively implement breakthrough digital technologies like AI are 12% more expensive and 26% more profitable than their competitors.
The development and implementation of artificial intelligence (AI) technology, and its successor - dataset collection, has become the very real technological revolution.
Scientists, enterprises, and entire research and analysis centers agree that off-the-shelf AI is one of the most important technological breakthroughs in human history, comparable to the invention of the printing press, steam engines, and electricity.
It's more or less clear: It's modern digital technology that largely determines which enterprises will survive and which will fall by the wayside. Many enterprises have already realized that now is the ideal time to implement AI solutions, given that they can not only help optimize business processes but also potentially provide access to new sources of revenue.
We have prepared tips for enterprises that are thinking about AI implementation.
Tip 1: Determine AI purpose
Many enterprises have high expectations of AI not always understanding the ultimate goal of implementing such sophisticated technology. The first thing to do is to identify the problem you would like to solve with AI.
Enterprize should have a clear and compelling business case for how AI will help it. If you can't clearly define the problem, you shouldn't even start AI projects. It's worth noting that AI is not suitable for all problems. Some of them can be better solved by traditional computing combined with good analytics.
Once you've identified the problem, it's important to thoughtfully create a strategy and action plan for implementing AI. It sounds easy, but it's a complicated process. "AI should be a strategy, not a solution," said Christian Klap, CEO at IvoryResearch.
Tip 2: Choose the right AI tools
Of course, the AI ecosystem is incredibly complex and confusing, which entails the need to retrain or hire new employees. Certain skills are also required to effectively manage projects and processes, which is why marketing and technical departments begin to work together in harmonious tandem during the implementation phase of AI products.
But it is important to choose the right platform to work effectively on AI implementation and optimization.
The rapid growth of artificial intelligence and machine programming technologies and their increasing proliferation across virtually all industries has spawned a plethora of technical and consulting opportunities, ranging from free, open-source "home-made" software to software as a service (SaaS) and customized turnkey solutions.
Because the AI ecosystem is evolving so rapidly, it's not easy to optimize. Many competing AI companies offer similar products and services as well as interchangeable ones. Today there are several software AI types, as well as opportunities to control them.
First, these are so-called low-level, open-source machine learning frameworks that provide the infrastructure for AI modeling. They allow custom algorithms to be configured to solve complex problems.
Popular examples of these frameworks are TensorFlow, PyTorch, MXNet, Caffe, Theano, Microsoft Cognitive Toolkit (CNTK), Keras, and Chainer. Each of them is used for different purposes and has its pros and cons. For example, TensorFlow is great for voice, text, and image recognition, while Chainer is often used for machine translation, speech recognition, and sentiment analysis.
While these frameworks provide great flexibility for many tasks, they also require the direct involvement of machine learning engineers.
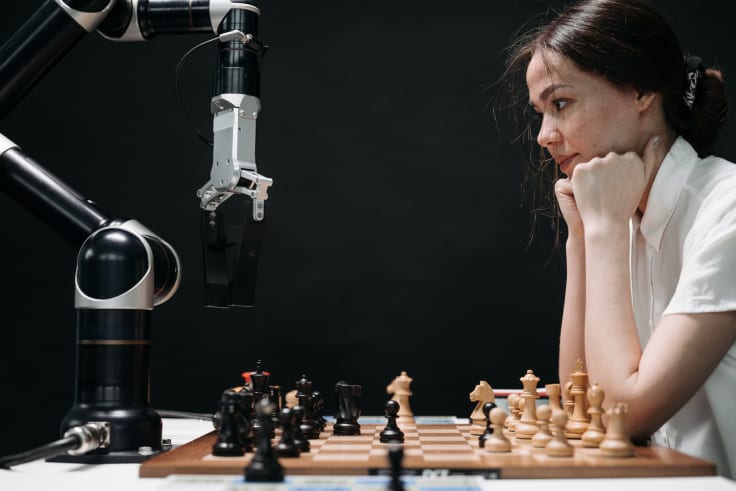
There are also higher-level commercial applications that are built on machine learning platforms and provide additional functionality and application programming interfaces (APIs). As a rule, these technologies are used to connect with user systems.
These technologies include IBM Watson, Google NLP, Microsoft Cognitive Services, as well as SaaS products and embedded AI from cloud providers such as SAP, Adobe, Salesforce, and Emarsys. These services are designed to help participants work quickly with AI.
This level of software helps solve several key problems and eliminates the need for intensive programming and direct data handling.
There are also turnkey solutions that are customized to solve specific industry or category problems. Providers of these services help their clients in the end-to-end implementation process. This option is suitable for a quick AI implementation process without creating a model from scratch.
Tip 3: Assemble a competent team of experts
Assembling an interdisciplinary team to support an AI project is another important challenge for enterprises. Team selection is part of a massive digital transformation within the organization.
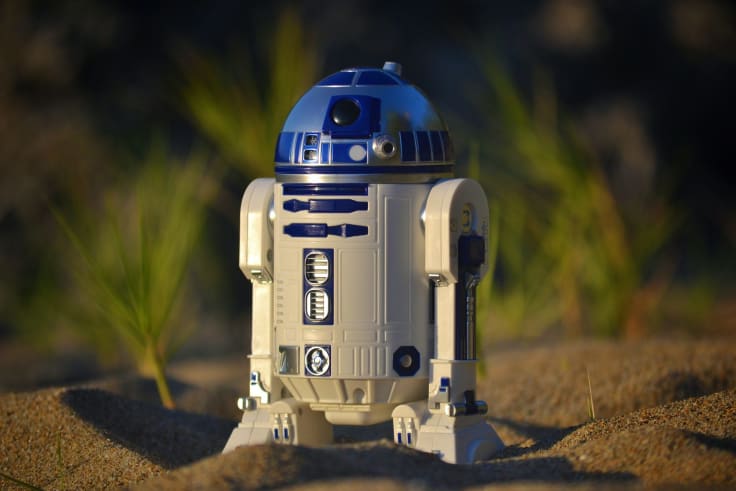
Unfortunately, assembling the perfect AI project team isn't always easy. First, new roles may need to be defined for existing employees in the organization, some of whom may need to be replaced or retrained with new skills. Second, hiring AI experts is complicated by a complex current shortage of skilled labor.
The PwC study found that 60% of executives at U.S. corporations using or researching AI planned to implement continuous training initiatives for AI employees. 47% planned to change their productivity and development structure to include AI skills. 44% planned to expand talent acquisition by implementing internships and developing partnerships with universities.
Tip 4: Improve artificial intelligence technology
The last best tip involves making sure artificial intelligence systems are constantly improving, measuring, and analyzing them over time. A "set it and forget it" strategy doesn't work here.
Artificial intelligence systems help and enhance human performance, and require detailed controls and algorithm updates to ensure there is no margin for error and no influence from fraudulent actions.
While there is no right way to measure AI performance, enterprises are experimenting with different models for measuring AI success.
Conclusion
What factors should enterprises consider before implementing AI? First, the enterprise should have a clear idea of how AI will solve a particular problem. The decision to build or buy off-the-shelf AI solutions depends on the availability of resources, the problems to be solved, and the culture of interaction in the enterprise.
Enterprises must also be willing to invest in improving and adapting systems. This will help avoid unnecessary business risks that conflict with regulatory requirements or violate general codes of ethics.
If you doubt whether you should implement the latest technology or not, remember - in the future, success will come to those who can achieve maximum results with minimum costs. And the introduction of artificial intelligence is one of the most effective tools.